How CML Optimization Prioritizes in Newton™
In heavy-process industries, facilities do all they can to minimize risk. They encounter risks associated with production loss and, more importantly, safety. For fixed equipment, we typically manage risk by performing routine inspections across multiple condition monitoring locations (CMLs) in order to monitor degradation rates, quantify damage states, and detect problems before they occur. Subject Matter Experts (SMEs) are used to select potential locations, but, by nature, can be very conservative due to industry standards and the high consequences of an error. This can result in a very high number of CMLs and significant cost to maintain these programs.
To solve this problem, we have developed a data-driven approach to CML optimization, which quantitatively optimizes inspection scope and intervals based on historical inspection data and subject matter expert input. Leveraging Newton™, our quantitative analytics platform, our machine learning models combine expected SME-assigned corrosion rates and observed CML thickness measurements to project future degradation and the associated uncertainty for each CML. This enables you to identify which CMLs account for the largest amounts of risk and potential production loss over time. The application identifies not only CMLs with low estimated remaining life but also identifies gaps where more data is needed to minimize the unknown, overall to optimize risk management.
What Makes Newton™ Different?
CML Optimization in Newton sets itself apart from other technology in the industry by providing:
- No subjectivity
- Quantitative value proposition
- A consistent and scalable approach
- The combined power of data with SME guidance
- An entire methodology based on reducing uncertainty & how each reading contributes to future uncertainty
- An evaluation of all CMLs to get statistical model of the entire circuit
- Projected performance based on Availability and not just risk
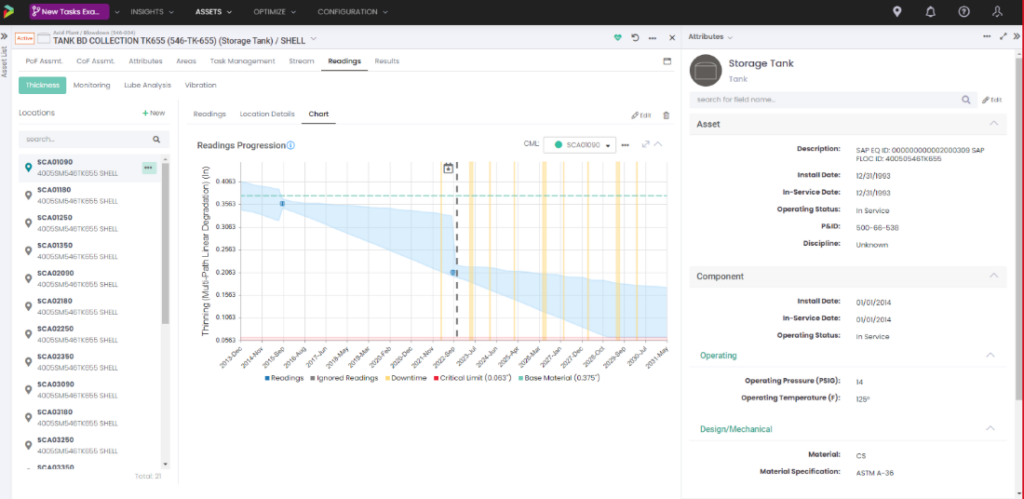
Quantitative Reliability Optimization (QRO) Example Application: CML Optimization
For more information about how Newton™ can be leveraged for CML optimization, read our white paper.
Stay in the know.
Providing data-driven insights, perspectives, and industrial inspiration from the forefront of the reliability transformation.