4 Machine Learning Solutions That Help Fill Data Gaps
Reliability is the key to making sure that a facility operates safely, and often, complex process facilities are plagued with missing or unreliable data. Data-driven reliability frameworks are focused on using traditional reliability methods in conjunction with machine learning and data science to give operators confidence in their strategic business decisions.
Machine Learning is the term used to describe a software’s ability to recognize patterns and learn and predict from those patterns. Machine Learning builds algorithms based on sample or past data, known as training data, to build parameters that identify a type of event without explicitly being programmed to do so. Machine Learning makes it possible to analyze past data and conditions to recognize when a process is abnormal vs normal.
This technology is useful in a reliability setting as machine learning can be used to analyze the environment that surrounded past failures. With that information, parameters can be set to help identify and alert users when those factors arise to help prevent additional failures in the future.
First, facilities must identify what is the right data and how you can use the data you’re currently collecting to implement more effective data groundwork at your facility. The use of data science and machine learning provides the ability to analyze existing data to intelligently identify and fill in gaps making the most out of the information you already have. These are four solutions rooted in machine learning that can help you optimize the data you already have:
1. Quantitative Reliability Optimization (QRO)
QRO merges traditional Risk Based Inspection (RBI), Reliability Centered Maintenance (RCM), and Reliability Availability Maintainability (RAM) programs with machine learning to create a dynamic reliability model. QRO applies data science principals to bridge gaps between historically siloed programs within a facility, enables reliability and operations leaders to optimize and make strategic reliability decisions. QRO is broken into the four elements below:
- Asset Risk Analysis (ARA)
- Lifetime Variability Curve (LVC)
- Forecasting System Availability and Throughput Modeling
- Reliability Simulation and Performance Optimization
2. Condition Monitoring Location (CML) Prioritization
Historically, CML Optimization has been focused on making sure facilities have effective and efficient CML coverage. With the use of data science, Pinnacle has developed a new methodology called CML Prioritization that analyzes the existing CMLs that have been placed rather than adding or removing them. CML Prioritization assesses the value of inspecting each CML using Lifetime Variability Curves (LVCs) to determine a window of time when it should be next inspected or replaced.
One aspect of this analysis is a concept called CML Families, where CMLs are grouped by similar physical and corrosion characteristics such as component type, process, and temperature. CML Families allow for thinning data to be shared across its members, creating the ability to replace corrosion model assumptions as a factor in initial rates. Learn how this methodology was used to help a North American facility reduce risk and identify minimum reduced inspection spend of $384K.
3. Unit Studies
Machine Learning techniques can be leveraged to create an automated, data-driven model that predicts degradation rates for assets and their components. This type of model utilizes process and operations data, as well as other information about the asset, to learn how to predict more realistic degradation rates in place of industry rule-based models, such as those included in API, NACE and other industry sources.
Pinnacle has conducted analysis that compares the accuracy predicted by their machine learning model versus the degradation rates predicted by a subject matter expert using current industry standards. Read how a machine learning model provided significantly more accurate predictions than standard industry practices in this Reformer Study White Paper.
4. Automated Inspection Grading
Inspection grading is an important pillar in an RBI program to ensure that a facility is getting proper credit for its inspections and risk is updated accordingly. Although this is not a traditional “not enough data” issue, it is an area that can be timely, expensive, and prone to human error. Pinnacle has developed a method to leverage machine learning to optimize and automate the grading process. An added benefit of automated grading is improved reliability by using a consistent set of rules.
In many cases, inspection reports are scanned documents or PDFs that make it difficult to extract information. That is where Optical Character Recognition (OCR) comes in. After the information is extracted, algorithms and machine learning are leveraged to assign grades according to custom grading criterion for your facility.
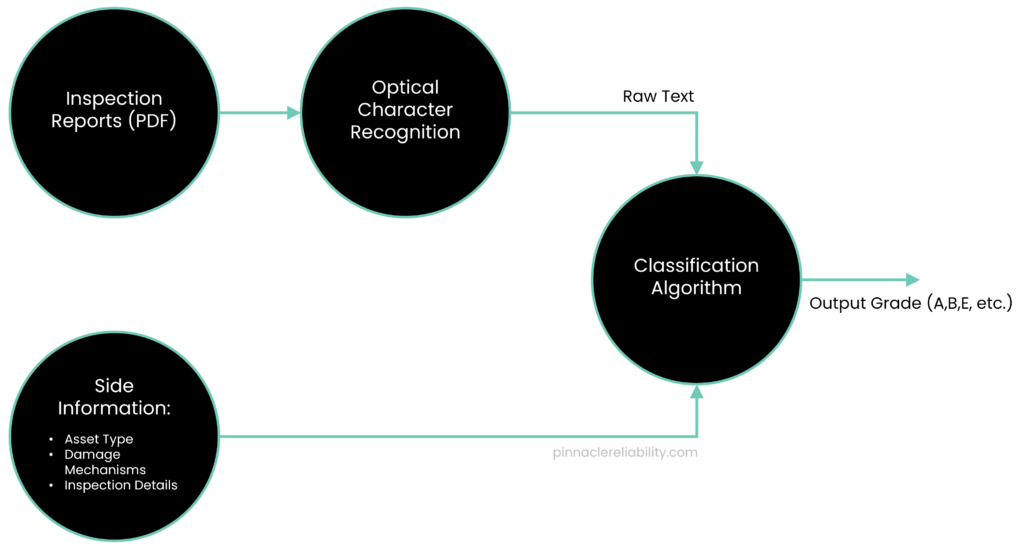
Stay in the know.
Providing data-driven insights, perspectives, and industrial inspiration from the forefront of the reliability transformation.