Learn how Pinnacle leveraged image analytics to reduce costs and create staffing efficiency for several North American midstream and downstream operators.
Challenge
Several of Pinnacle’s customers were looking to perform external visual inspections faster and with fewer resources while not compromising quality.
Solution
Pinnacle teamed up with SoftServe to implement image analytics across several sites to complete a Proof of Concept (POC) for the new methodology to create efficiencies and improve inspection planning.
Result
The POC proved that image analytics can reduce waste and inspection spend by reducing the overall variability and uncertainty in external corrosion due to human subjectivity in inspections.
The Challenge
Corrosion management and asset inspections play a vital role in the safety and reliability of a facility and are key factors in financial planning. According to the Asset Integrity Management Global Market Report 2022, Mechanical Integrity (MI) costs are estimated to exceed $25B for 2022. Emphasizing the need for cost-effective and robust corrosion assessment and mitigation approaches is vital to preventing Loss of Primary Containment (LOPC) failures and unplanned downtime.
Changing the inspection process from reactive to proactive corrosion management is one solution, but it increases the amount of data collected, processed, and interpreted. Adopting Artificial Intelligence (AI), robotics, and other emerging technologies is becoming increasingly essential to creating efficiencies in this process.
Pinnacle's Solution
For this POC, Pinnacle partnered with SoftServe to build the computer vision algorithms needed to investigate how panoramic or 360-degree handheld images with corrosion labels can be used to train a ML model to automatically detect corrosion and calculate the percentage of corrosion coverage based on an object’s image.
Pinnacle chose to pilot this approach simultaneously with two different customers. Each pilot was broken into five phases.

Phase 1 Photo Collection
Pinnacle collected images and videos in the field using a Ricoh Theta Z1 360-degree camera. Equirectangular images with high resolution were gathered to conduct the analysis. Equirectangular images are single images stitched together from a 360-degree horizontal and 180-degree vertical view.
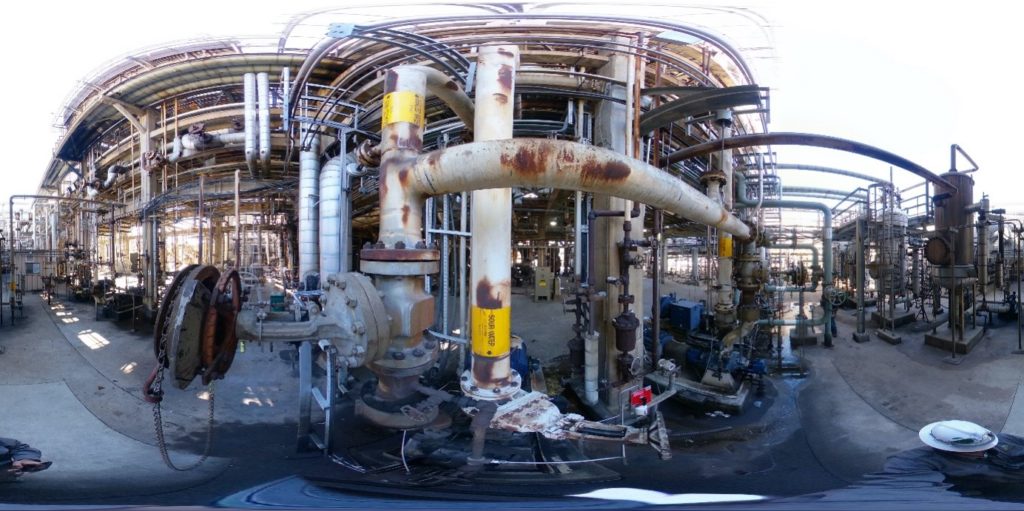
Phase 2 Photo Preparation
Equirectangular images are complicated both for labeling and ML model training. Labeling includes highlighting or identifying a specific part of an image for training in the ML model. Also, it is worth having a model for non-panoramic images for further perspective as it will be more usable for other data sources.
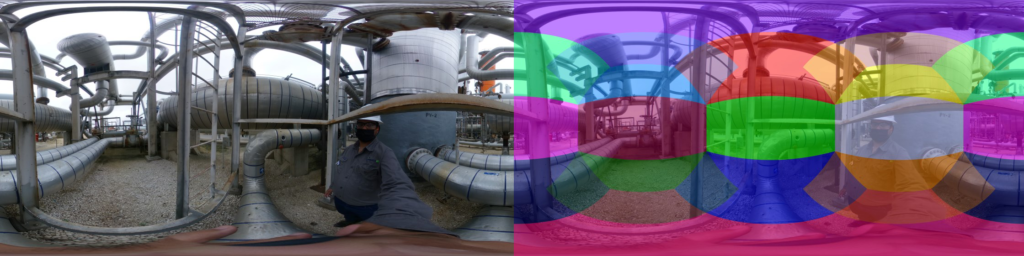
Phase 3 Corrosion Detection
Equirectangular images are complicated both for labeling and ML model training. Labeling includes highlighting or identifying a specific part of an image for training in the ML model. Also, it is worth having a model for non-panoramic images for further perspective as it will be more usable for other data sources.
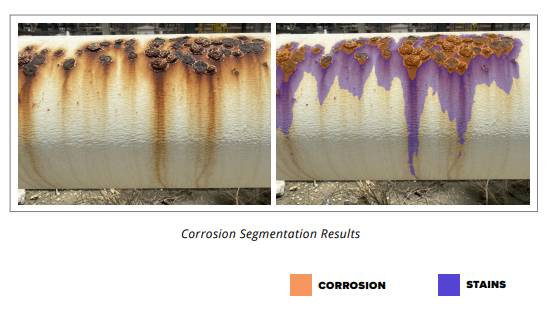
Phases 4 & 5 Report and Results Preparation and Feedback
Equirectangular images are complicated both for labeling and ML model training. Labeling includes highlighting or identifying a specific part of an image for training in the ML model. Also, it is worth having a model for non-panoramic images for further perspective as it will be more usable for other data sources.
Results
In the traditional way of conducting an inspection, an API 570 inspector would use a work package to inspect a particular pipe segment. Still, as they go out to inspect, they may see multiple issues in the surrounding area. Because of the systems in place, that inspector most likely will not document the problems they see and will only write a report for the assigned piping segment. Typically, additional reporting will only happen if something significant is observed, such as a leak.
With the solution described above, the cameras and computers capture everything in the frame, not just the pipe being inspected. An inspector can capture multiple assets simultaneously and perform a basic screening in one click. From there, an SME can evaluate it to pinpoint precisely what assets need additional attention. Other important aspects to highlight are:
- Screening can be conducted on an entire plant in just a matter of days, depending on plant size.
- Personnel can capture the images in just a fraction of the time it would take to do planned inspections.
- This methodology can be used to screen hard to reach areas with ease, such as pipe racks and high- temperature assets that would normally require shutdown or scaffolding to be built.
This POC created a heat map to identify areas of interest. It pulls up the associated image along with your GPS coordinates and gives a graph percentage of what kind of damage it sees. Computer imaging software and image analytics help eliminate human subjectivity. For example, an inspector may go into the field and make a recommendation to code align to a damage mechanism. Still, even when missing paint and light surface oxidation is observed during an SME review, the SME’s recommendation may be not to do anything.
Throughout the POC, SMEs validated the results, and in many cases, they agreed with the corrosion prediction from the computer vision models. Over 75% of the time, SMEs said that the results from ML algorithms met their expectations for external corrosion detection. Almost 50% of the time, the SMEs didn’t agree with each other, such as an inspector recommending coating replacement for low instances of corrosion and coating breakdown, but another SME disagrees with that recommendation. This leads us to believe that such a solution can help us standardize corrosion detection and drive the right actions to optimize cost and resources. In this case, accuracy is defined as what a human would identify as corrosion, and the computer was able to identify it as well. At the end of these POC projects, each customer was provided with the following deliverables:
- Corrosion Segmentation Model- outlines the detection of corrosion and rust stains on images of the facilities taken by a panoramic camera.
- Texture Classification ML Model- outlines the detection of paint defects, rust staining, light, and severe corrosion on close-up images of the stationary equipment.
- User interface (UI)- browser application hosted on the secured Virtual Machine (VM), allowing users to tune processing parameters, run ML models in real-time on the selected images, and see the big picture on the map. The UI enables the user to process images one by one with a preview or batch processing of the entire directory passed as a parameter.
- Technical Report- the report outlines the chosen approaches and techniques for building the pipeline and incorporates the results of the main experiments with the conclusions provided and potential improvements for future development.
Conclusion
With the completion of this POC, Pinnacle has proved that image analytics can reduce waste and overall costs by reducing the overall variability and uncertainty in external corrosion due to human subjectivity in inspections. Another benefit to this methodology is that it can be used at any level of the plant including a full plant-wide assessment. The next phase of this POC is to leverage a similar solution for Thermal Corrosion Under Insulation (CUI) anomaly screening, structural anomalies, and other advanced screening methods.
The overall goal of this methodology is to aid SMEs and plant managers in optimizing their field resources to get repeatable, reliable data without subjectivity. Eventually, Pinnacle aims to complete an overall external 510/570 governed API inspection for an entire unit, such as a crude unit, within a two-to-three-week period.
Contact us to speak to one of our experts about how Pinnacle can help evolve your inspection program.
Stay in the know.
Providing data-driven insights, perspectives, and industrial inspiration from the forefront of the reliability transformation.